Maximizing Efficiency with Image Labeling: The Ultimate Data Annotation Guide
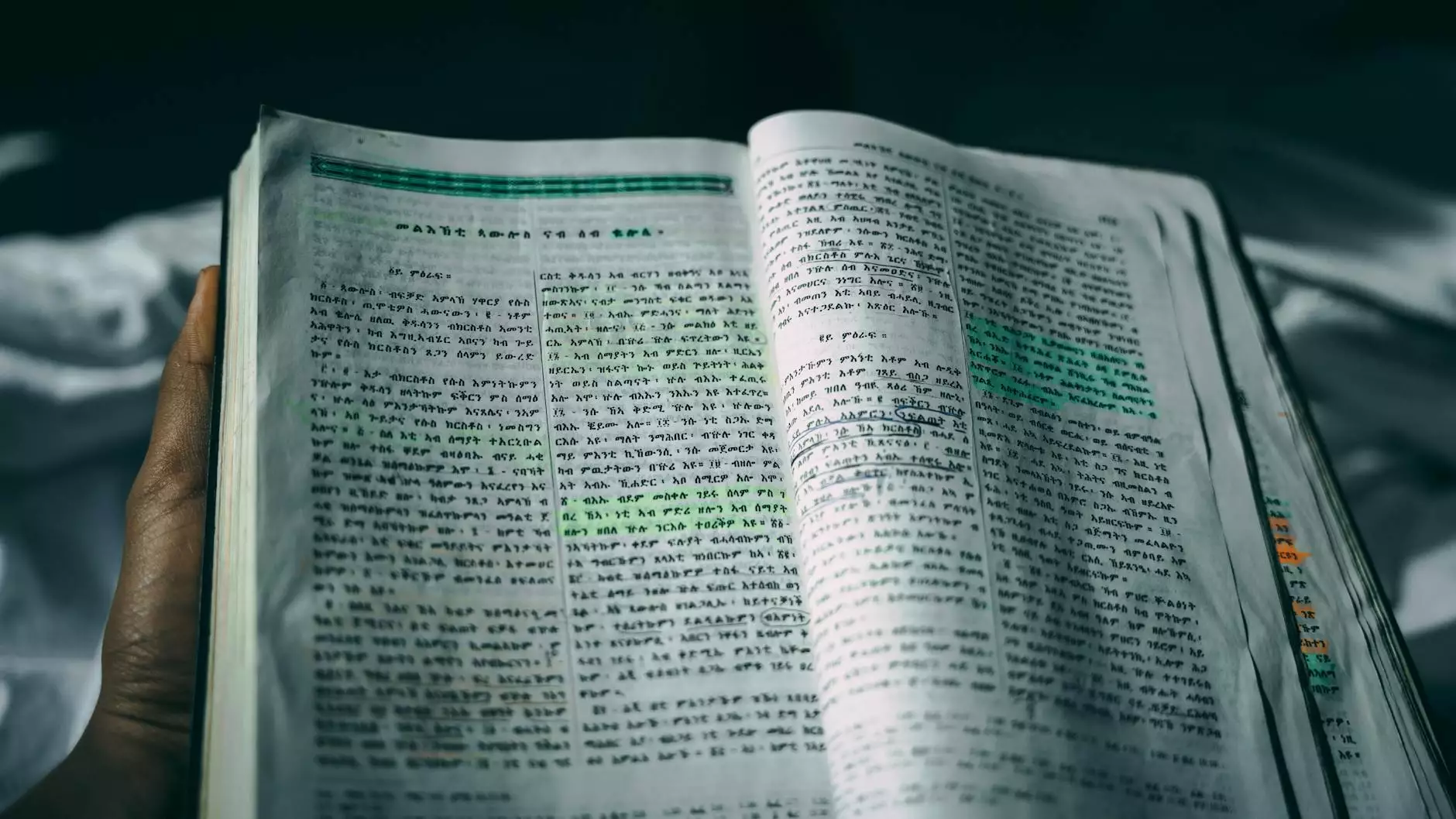
In today's digital landscape, the demand for high-quality data is at an all-time high. Companies across various industries are leveraging data annotation to train machine learning models, improve AI algorithms, and enhance user experiences. Among the various forms of data annotation, image labeling stands out as one of the most crucial components. This comprehensive guide delves into the significance, benefits, and methodologies of image labeling using keylabs.ai's data annotation platform.
Understanding Image Labeling
Image labeling refers to the process of tagging or annotating images with specific labels that describe the content of those images. This is a foundational step in training machine learning models, especially in computer vision applications. Without accurate image labeling, AI systems cannot learn effectively, leading to poor performance and results.
The Importance of Image Labeling in Machine Learning
For machine learning algorithms to understand and interpret visual data, they require labeled datasets. Here are the key reasons why image labeling is critical:
- Accuracy: Well-labeled images improve the accuracy of AI models, ensuring they make correct predictions.
- Data Quality: High-quality annotated data leads to better model performance.
- Scalability: Automated image labeling processes enable businesses to scale their machine learning initiatives.
- Variety of Applications: Image labeling is vital in various applications, such as facial recognition, self-driving cars, medical imaging, and more.
Types of Image Labeling Techniques
There are several techniques used in image labeling, each with its unique applications and advantages. Understanding these techniques can help businesses choose the right approach for their data needs:
1. Bounding Boxes
Bounding boxes are rectangular boxes that are drawn around objects in an image. This technique is widely used for object detection tasks.
2. Polygonal Segmentation
This technique involves outlining the exact shape of an object in an image. It provides more accuracy than bounding boxes, making it ideal for complex objects.
3. Semantic Segmentation
Semantic segmentation assigns a class label to every pixel in an image. This technique is crucial for tasks where precise object delineation is needed, such as in medical imaging.
4. Instance Segmentation
Instance segmentation is an advanced form of segmentation that differentiates between separate instances of the same object. This is particularly useful in crowded scenes.
The Role of Data Annotation Tools and Platforms
Data annotation tools facilitate the process of image labeling, making it faster and more efficient. keylabs.ai offers a robust data annotation platform designed to meet the needs of businesses engaging in machine learning projects. Here’s how it enhances the labeling process:
- User-Friendly Interface: Intuitive design allows annotators to work efficiently without extensive training.
- Collaboration Features: Teams can work together seamlessly on projects, ensuring consistency and accuracy in annotations.
- Advanced Automation: Automated labeling tools reduce the workload on human annotators and improve turnaround times.
- Quality Assurance: Built-in quality checks ensure that image labeling meets high standards before datasets are finalized.
Steps Involved in Image Labeling
Effective image labeling involves a series of steps that ensure the resulting data is reliable and useful. Here’s a breakdown of the typical workflow:
Step 1: Define the Objectives
Before starting the labeling process, it’s essential to outline the goals of the project. What will the labeled images be used for? This clarity will guide the entire annotation process.
Step 2: Select a Data Annotation Tool
Choosing the right tool, like keylabs.ai, is crucial. Consider factors such as ease of use, automation capabilities, and support for various labeling techniques.
Step 3: Data Preparation
Gather the images that will be labeled. Ensure that they are in a suitable format and size for the chosen annotation tool.
Step 4: Annotation Process
Begin the labeling process. Depending on the chosen technique, use bounding boxes, polygons, or segmentation to annotate the images. Make sure to maintain consistency throughout the dataset.
Step 5: Quality Control
After labeling, review the annotations for accuracy and consistency. Utilize quality control tools offered by platforms like keylabs.ai to enhance this phase.
Step 6: Export the Labeled Data
Once the labeling is complete and quality-checked, export the data in the required format for training machine learning models.
Challenges in Image Labeling and How to Overcome Them
While image labeling is essential for machine learning, it comes with its challenges. Here are some common hurdles and strategies to overcome them:
1. Scalability
As projects grow, the volume of images requiring labeling can become overwhelming.
- Solution: Utilize platforms that offer automation to speed up the annotation process, allowing teams to handle larger datasets efficiently.
2. Consistency
Maintaining consistency across different annotators can be challenging, especially in large teams.
- Solution: Implement comprehensive annotation guidelines and use tools that include consensus checks to ensure uniformity.
3. Quality Assurance
The quality of annotations can vary, affecting the performance of AI models.
- Solution: Regularly review labeled data and implement a quality control steps, such as peer reviews and automated validation.
The Future of Image Labeling
As artificial intelligence continues to evolve and shape our world, the demand for image labeling will only increase. Innovations in data annotation tools will likely include:
- Enhanced Automation: Increased use of AI and machine learning to automate more aspects of the labeling process, reducing time and costs.
- Integration with AI Models: Direct integration of labeling tools with machine learning pipelines for real-time data annotation.
- Improved Collaboration: Features fostering collaboration across teams and organizations, allowing for shared datasets and projects.
Conclusion
In conclusion, image labeling is a fundamental aspect of data annotation that enables machine learning models to accurately understand images. Platforms like keylabs.ai provide businesses with the tools necessary to streamline this process, ensuring high-quality data is produced efficiently. By embracing effective image labeling practices, companies can maximize the performance of their AI initiatives and stay ahead in an increasingly competitive landscape.
As we move forward, investing in robust annotation tools and adhering to best practices will be crucial. The world of data is rapidly evolving, and those who prioritize accurate and efficient labeling will undoubtedly reap the benefits in their AI projects.